报告内容:Moment-based distributionally robust optimization (DRO) provides an optimization framework to integrate statistical information with traditional optimization approaches. Under this framework, one assumes that the underlying joint distribution of random parameters runs in a distributional ambiguity set constructed by moment information and makes decisions against the worst-case distribution within the set. Although most moment-based DRO problems can be reformulated as semidefinite programming (SDP) problems that can be solved in polynomial time, solving high-dimensional SDPs is still time-consuming. Unlike existing approximation approaches that first reduce the dimensionality of random parameters and then solve the approximated SDPs, we propose an optimized dimensionality reduction (ODR) approach. We first show that the ranks of the matrices in the SDP reformulations are small, by which we are then motivated to integrate the dimensionality reduction of random parameters with the subsequent optimization problems. Such integration enables two outer and one inner approximations of the original problem, all of which are low-dimensional SDPs that can be solved efficiently, providing two lower bounds and one upper bound correspondingly. More importantly, these approximations can theoretically achieve the optimal value of the original high-dimensional SDPs. As these approximations are nonconvex SDPs, we develop modified Alternating Direction Method of Multipliers (ADMM) algorithms to solve them efficiently. We demonstrate the effectiveness of our proposed ODR approach and algorithm in solving multiproduct newsvendor and production-transportation problems. Numerical results show significant advantages of our approach on the computational time and solution quality over the three best possible benchmark approaches. Our approach can obtain an optimal or near-optimal (mostly within 0.1%) solution and reduce the computational time by up to three orders of magnitude.
报告人简介: 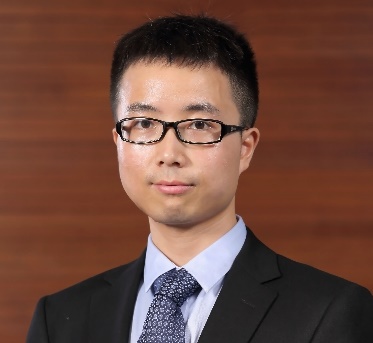
Kai Pan is currently an Associate Professor in Operations Management at the Faculty of Business of The Hong Kong Polytechnic University and the Director of the MSc Program in Operations Management. He received his Ph.D. degree in Industrial and Systems Engineering from the University of Florida in 2016 and his Bachelor's degree in Industrial Engineering from Zhejiang University in 2010. Previously, he worked as a Research Scientist at Amazon (Seattle, Washington) on Supply Chain Optimization and a Power System Engineer at GE Grid Solutions (Redmond, Washington) on Electricity Market Operations. His research interests include Stochastic and Discrete Optimization, Robust and Data-Driven Optimization, Dynamic Programming, and their applications in Energy Market, Smart City, Supply Chain, Shared Mobility, and Transportation. His research on these topics has been published in Operations Research, Manufacturing and Service Operations Management, INFORMS Journal on Computing, Production and Operations Management, IISE Transactions, European Journal of Operational Research, IEEE Transactions on Power Systems, Transportation Research Part B, etc. He was the first-place winner of the IISE Pritsker Doctoral Dissertation Award in 2017.
|